Understanding Semantic Analysis NLP

Semantic analysis is key to contextualization that helps disambiguate language data so text-based NLP applications can be more accurate. Google incorporated ‘semantic analysis’ into its framework by developing its tool to understand and improve user searches. The Hummingbird algorithm was formed in 2013 and helps analyze user intentions as and when they use the google search engine. As a result of Hummingbird, results are shortlisted based on the ‘semantic’ relevance of the keywords. Moreover, it also plays a crucial role in offering SEO benefits to the company.
In this example, LSA is applied to a set of documents after creating a TF-IDF representation. The resulting LSA model is used to print the topics and transform the documents into the LSA space. To know the meaning of Orange in a sentence, we need to know the words around it. We will evaluate our model using various metrics such as Accuracy Score, Precision Score, Recall Score, Confusion Matrix and create a roc curve to visualize how our model performed. Now, we will read the test data and perform the same transformations we did on training data and finally evaluate the model on its predictions.
Semantic Analysis is a topic of NLP which is explained on the GeeksforGeeks blog. The entities involved in this text, along with their relationships, are shown below. Hence, under Compositional Semantics Analysis, we try to understand how combinations of individual words form the meaning of the text. The visual aspect is easier for users to navigate and helps them see the larger picture. The search results will be a mix of all the options since there is no additional context.
What Semantic Analysis Means to Natural Language Processing
As we discussed, the most important task of semantic analysis is to find the proper meaning of the sentence. You see, the word on its own matters less, and the words surrounding it matter more for the interpretation. A semantic analysis algorithm needs to be trained with a larger corpus of data to perform better. Syntactic analysis involves analyzing the grammatical syntax of a sentence to understand its meaning. In this article, we will focus on the sentiment analysis using NLP of text data.
Additionally, it delves into the contextual understanding and relationships between linguistic elements, enabling a deeper comprehension of textual content. In AI and machine learning, semantic analysis helps in feature extraction, sentiment analysis, and understanding relationships in data, which enhances the performance of models. The first is lexical semantics, the study of the meaning of individual words and their relationships. This stage entails obtaining the dictionary definition of the words in the text, parsing each word/element to determine individual functions and properties, and designating a grammatical role for each. Key aspects of lexical semantics include identifying word senses, synonyms, antonyms, hyponyms, hypernyms, and morphology. In the next step, individual words can be combined into a sentence and parsed to establish relationships, understand syntactic structure, and provide meaning.
Top 15 sentiment analysis tools to consider in 2024 – Sprout Social
Top 15 sentiment analysis tools to consider in 2024.
Posted: Tue, 16 Jan 2024 08:00:00 GMT [source]
All in all, semantic analysis enables chatbots to focus on user needs and address their queries in lesser time and lower cost. Semantic analysis methods will provide companies the ability to understand the meaning of the text and achieve comprehension and communication levels that are at par with humans. Semantic analysis plays a vital role in the automated handling of customer grievances, managing customer support tickets, and dealing with chats and direct messages via chatbots or call bots, among other tasks. The semantic analysis uses two distinct techniques to obtain information from text or corpus of data. The first technique refers to text classification, while the second relates to text extractor.
Critical elements of semantic analysis
Efficiently working behind the scenes, semantic analysis excels in understanding language and inferring intentions, emotions, and context. MonkeyLearn makes it simple for you to get started with automated semantic analysis tools. Using a low-code UI, you can create models to automatically analyze your text for semantics and perform techniques like sentiment and topic analysis, or keyword extraction, in just a few simple steps. The first part of semantic analysis, studying the meaning of individual words is called lexical semantics. It includes words, sub-words, affixes (sub-units), compound words and phrases also.
By comprehending the intricate semantic relationships between words and phrases, we can unlock a wealth of information and significantly enhance a wide range of NLP applications. In this comprehensive article, we will embark on a captivating journey into the realm of semantic analysis. We will delve into its core concepts, explore powerful techniques, and demonstrate their practical implementation through illuminating code examples using the Python programming language. Get ready to unravel the power of semantic analysis and unlock the true potential of your text data. Semantics gives a deeper understanding of the text in sources such as a blog post, comments in a forum, documents, group chat applications, chatbots, etc. With lexical semantics, the study of word meanings, semantic analysis provides a deeper understanding of unstructured text.
With growing NLP and NLU solutions across industries, deriving insights from such unleveraged data will only add value to the enterprises. For example, semantic analysis can generate a repository of the most common customer inquiries and then decide how to address or respond to them. For example, ‘Raspberry Pi’ can refer to a fruit, a single-board computer, or even a company (UK-based foundation). Hence, it is critical to identify which meaning suits the word depending on its usage. So, mind mapping allows users to zero in on the data that matters most to their application.
- It could be BOTs that act as doorkeepers or even on-site semantic search engines.
- Currently, there are several variations of the BERT pre-trained language model, including BlueBERT, BioBERT, and PubMedBERT, that have applied to BioNER tasks.
- The resulting LSA model is used to print the topics and transform the documents into the LSA space.
- One can train machines to make near-accurate predictions by providing text samples as input to semantically-enhanced ML algorithms.
It’s an essential sub-task of Natural Language Processing (NLP) and the driving force behind machine learning tools like chatbots, search engines, and text analysis. However, machines first need to be trained to make sense of human language and understand the context in which words are used; otherwise, they might misinterpret the word “joke” as positive. Now, we can understand that meaning representation shows how to put together semantic analysis nlp the building blocks of semantic systems. In other words, it shows how to put together entities, concepts, relation and predicates to describe a situation. But before getting into the concept and approaches related to meaning representation, we need to understand the building blocks of semantic system. It is the first part of the semantic analysis in which the study of the meaning of individual words is performed.
The accuracy of the summary depends on a machine’s ability to understand language data. This is a key concern for NLP practitioners responsible for the ROI and accuracy of their NLP programs. You can proactively get ahead of NLP problems by improving machine language understanding. It recreates a crucial role in enhancing the understanding of data for machine learning models, thereby making them capable of reasoning and understanding context more effectively. Semantic analysis forms the backbone of many NLP tasks, enabling machines to understand and process language more effectively, leading to improved machine translation, sentiment analysis, etc.
A company can scale up its customer communication by using semantic analysis-based tools. It could be BOTs that act as doorkeepers or even on-site semantic search engines. By allowing customers to “talk freely”, without binding up to a format – a firm can gather significant volumes of quality data. Natural Language Processing or NLP is a branch of computer science that deals with analyzing spoken and written language. Advances in NLP have led to breakthrough innovations such as chatbots, automated content creators, summarizers, and sentiment analyzers.
Now, let’s say you search for “cowboy boots.” Using semantic analysis, Google can connect the words “cowboy” and “boots” to realize you’re looking for a specific type of shoe. Jose Maria Guerrero, an AI specialist and author, is dedicated to overcoming that challenge and helping people better use semantic analysis in NLP. These tools enable computers (and, therefore, humans) to understand the overarching themes and sentiments in vast amounts of data. Tools like IBM Watson allow users to train, tune, and distribute models with generative AI and machine learning capabilities. NLP is the ability of computers to understand, analyze, and manipulate human language. With the help of meaning representation, we can link linguistic elements to non-linguistic elements.
Relationship extraction is a procedure used to determine the semantic relationship between words in a text. In semantic analysis, relationships include various entities, such as an individual’s name, place, company, designation, etc. Moreover, semantic categories such as, ‘is the chairman of,’ ‘main branch located a’’, ‘stays at,’ and others connect the above entities. Semantic analysis in NLP is the process of understanding the meaning and context of human language.
In this component, we combined the individual words to provide meaning in sentences. This article is part of an ongoing blog series on Natural Language Processing (NLP). I hope after reading that article you can understand the power of NLP in Artificial Intelligence. So, in this part of this series, we will start our discussion on Semantic analysis, which is a level of the NLP tasks, and see all the important terminologies or concepts in this analysis. Homonymy and polysemy deal with the closeness or relatedness of the senses between words. Homonymy deals with different meanings and polysemy deals with related meanings.
It is also a key component of several machine learning tools available today, such as search engines, chatbots, and text analysis software. NER is a key information extraction task in NLP for detecting and categorizing named entities, such as names, organizations, locations, events, etc.. NER uses machine learning algorithms trained on data sets with predefined entities to automatically analyze and extract entity-related information from new unstructured text. NER methods are classified as rule-based, statistical, machine learning, deep learning, and hybrid models.
They have created a website to sell their food and now the customers can order any food item from their website and they can provide reviews as well, like whether they liked the food or hated it. In a time overwhelmed by huge measures of computerized information, Chat PG understanding popular assessment and feeling has become progressively pivotal. This acquaintance fills in as a preliminary with investigate the complexities of feeling examination, from its crucial ideas to its down to earth applications and execution.

We will pass this as a parameter to GridSearchCV to train our random forest classifier model using all possible combinations of these parameters to find the best model. Now, we will convert the text data into vectors, by fitting and transforming the corpus that we have created. Because, without converting to lowercase, it will cause an issue when we will create vectors of these words, as two different vectors will be created for the same word which we don’t want to.
This step is termed ‘lexical semantics‘ and refers to fetching the dictionary definition for the words in the text. You can foun additiona information about ai customer service and artificial intelligence and NLP. Each element is designated a grammatical role, and the whole structure is processed to cut down on any confusion caused by ambiguous words having multiple meanings. Semantic analysis stands as the cornerstone in navigating the complexities of unstructured data, revolutionizing how computer science approaches language comprehension. Its prowess in both lexical semantics and syntactic analysis enables the extraction of invaluable insights from diverse sources. In semantic analysis, word sense disambiguation refers to an automated process of determining the sense or meaning of the word in a given context. As natural language consists of words with several meanings (polysemic), the objective here is to recognize the correct meaning based on its use.
Beyond just understanding words, it deciphers complex customer inquiries, unraveling the intent behind user searches and guiding customer service teams towards more effective responses. QuestionPro, a survey and research platform, might have certain features or functionalities that could complement or support the semantic analysis process. Thus, the ability of a machine to overcome the ambiguity involved in identifying the meaning of a word based on its usage and context is called Word Sense Disambiguation. With sentiment analysis, companies can gauge user intent, evaluate their experience, and accordingly plan on how to address their problems and execute advertising or marketing campaigns.
In other words, we can say that lexical semantics is the relationship between lexical items, meaning of sentences and syntax of sentence. Semantic analysis, also known as semantic parsing or computational semantics, is the process of extracting meaning from language by analyzing the relationships between words, phrases, and sentences. It goes beyond syntactic analysis, which focuses solely on grammar and structure. Semantic analysis aims to uncover the deeper meaning and intent behind the words used in communication.

Semantic analysis systems are used by more than just B2B and B2C companies to improve the customer experience. Moreover, while these are just a few areas where the analysis finds significant applications. Its potential reaches into numerous other domains where understanding language’s meaning and context is crucial. Chatbots, virtual assistants, and recommendation systems benefit from semantic analysis by providing more accurate and context-aware responses, thus significantly improving user satisfaction.
Moreover, it is also helpful to customers as the technology enhances the overall customer experience at different levels. While MindManager does not use AI or automation on its own, it does have applications in the AI world. For example, mind maps can help create structured documents that include project overviews, code, experiment https://chat.openai.com/ results, and marketing plans in one place. This technique is used separately or can be used along with one of the above methods to gain more valuable insights. For Example, Tagging Twitter mentions by sentiment to get a sense of how customers feel about your product and can identify unhappy customers in real-time.
In short, sentiment analysis can streamline and boost successful business strategies for enterprises. Chatbots help customers immensely as they facilitate shipping, answer queries, and also offer personalized guidance and input on how to proceed further. Moreover, some chatbots are equipped with emotional intelligence that recognizes the tone of the language and hidden sentiments, framing emotionally-relevant responses to them. Semantic analysis tech is highly beneficial for the customer service department of any company.
These chatbots act as semantic analysis tools that are enabled with keyword recognition and conversational capabilities. These tools help resolve customer problems in minimal time, thereby increasing customer satisfaction. Cdiscount, an online retailer of goods and services, uses semantic analysis to analyze and understand online customer reviews. When a user purchases an item on the ecommerce site, they can potentially give post-purchase feedback for their activity.
Learn How To Use Sentiment Analysis Tools in Zendesk
It is the first part of semantic analysis, in which we study the meaning of individual words. It involves words, sub-words, affixes (sub-units), compound words, and phrases also. Polysemy refers to a relationship between the meanings of words or phrases, although slightly different, and shares a common core meaning under elements of semantic analysis. Relationship extraction is the task of detecting the semantic relationships present in a text. Relationships usually involve two or more entities which can be names of people, places, company names, etc. These entities are connected through a semantic category such as works at, lives in, is the CEO of, headquartered at etc.
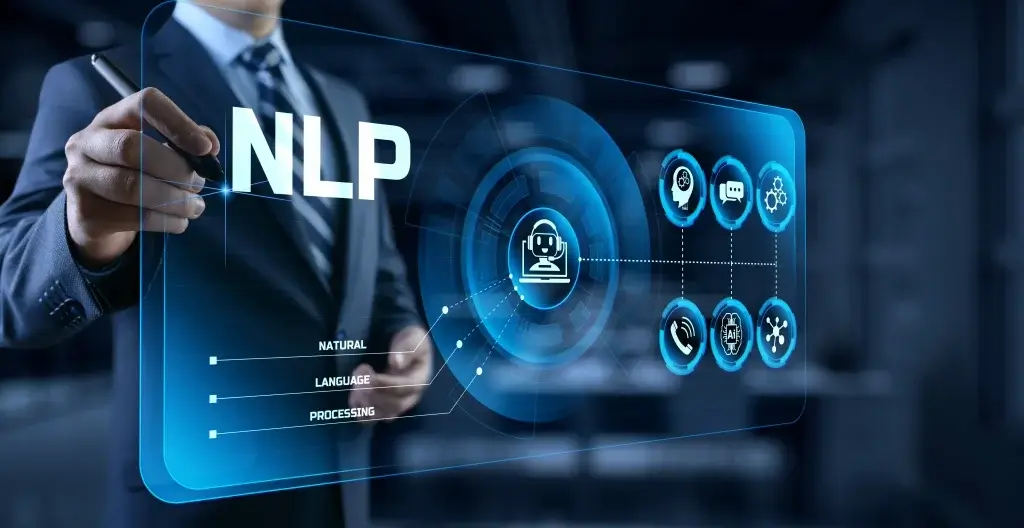
NER is widely used in various NLP applications, including information extraction, question answering, text summarization, and sentiment analysis. By accurately identifying and categorizing named entities, NER enables machines to gain a deeper understanding of text and extract relevant information. Driven by the analysis, tools emerge as pivotal assets in crafting customer-centric strategies and automating processes. Moreover, they don’t just parse text; they extract valuable information, discerning opposite meanings and extracting relationships between words.
Jose Maria Guerrero developed a technique that uses automation to turn the results from IBM Watson into mind maps. Trying to turn that data into actionable insights is complicated because there is too much data to get a good feel for the overarching sentiment. Antonyms refer to pairs of lexical terms that have contrasting meanings or words that have close to opposite meanings. Now, we will check for custom input as well and let our model identify the sentiment of the input statement. ‘ngram_range’ is a parameter, which we use to give importance to the combination of words, such as, “social media” has a different meaning than “social” and “media” separately. As the data is in text format, separated by semicolons and without column names, we will create the data frame with read_csv() and parameters as “delimiter” and “names”.

Sentiment analysis using NLP stands as a powerful tool in deciphering the complex landscape of human emotions embedded within textual data. As we conclude this journey through sentiment analysis, it becomes evident that its significance transcends industries, offering a lens through which we can better comprehend and navigate the digital realm. These challenges highlight the complexity of human language and communication. Overcoming them requires advanced NLP techniques, deep learning models, and a large amount of diverse and well-labelled training data. Despite these challenges, sentiment analysis continues to be a rapidly evolving field with vast potential.

For example, you might decide to create a strong knowledge base by identifying the most common customer inquiries. With the help of meaning representation, unambiguous, canonical forms can be represented at the lexical level. The main difference between them is that in polysemy, the meanings of the words are related but in homonymy, the meanings of the words are not related.
Semantic analysis, on the other hand, is crucial to achieving a high level of accuracy when analyzing text. Capturing the information is the easy part but understanding what is being said (and doing this at scale) is a whole different story. Semantic analysis employs various methods, but they all aim to comprehend the text’s meaning in a manner comparable to that of a human. This can entail figuring out the text’s primary ideas and themes and their connections.
Semantic analysis is an important subfield of linguistics, the systematic scientific investigation of the properties and characteristics of natural human language. It’s used extensively in NLP tasks like sentiment analysis, document summarization, machine translation, and question answering, thus showcasing its versatility and fundamental role in processing language. It’s not just about understanding text; it’s about inferring intent, unraveling emotions, and enabling machines to interpret human communication with remarkable accuracy and depth.
In conclusion, sentiment analysis is a powerful technique that allows us to analyze and understand the sentiment or opinion expressed in textual data. By utilizing Python and libraries such as TextBlob, we can easily perform sentiment analysis and gain valuable insights from the text. Whether it is analyzing customer reviews, social media posts, or any other form of text data, sentiment analysis can provide valuable information for decision-making and understanding public sentiment.